Wolfsberg Principles: How Complidata is using AI to reduce Financial Crime Risks
- Bharat Ram Ammu
- Mar 10, 2023
- 4 min read
Updated: Oct 18, 2024
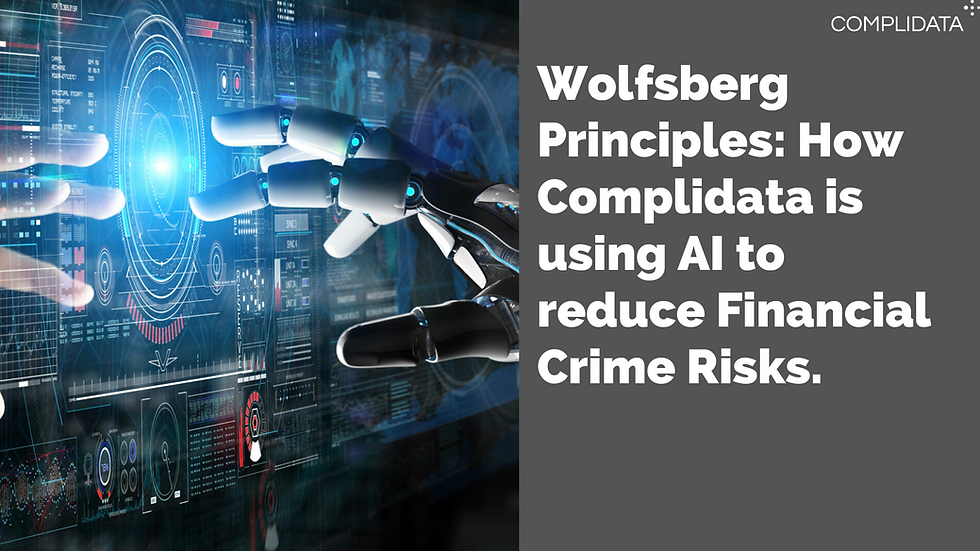
Recently, the Wolfsberg Group has published “Wolfsberg Principles for Using Artificial Intelligence and Machine Learning in Financial Crime Compliance”. The Principles, consisting of five elements (Legitimate Purpose, Proportionate Use, Design and Technical expertise, Accountability and Oversight, and Openness and Transparency), follow a risk-based approach, identifying several aspects of risk that could arise from the use of AI and Machine-learning in regulatory compliance processes, as well as guidance on how to tackle them.
At Complidata, we have developed our very own AI/ML Financial Crime Risk Reduction (FCRR) solutions, since 2018, with more than 20 projects delivered. In this article, we will look at how Complidata is applying closely the Principles to our product.
Legitimate Purpose:
When Complidata works with Financial Institutions (FIs) to reduce financial crime using Artificial Intelligence (AI), the data requirements are clear, relevant, and minimal. Working with clients, we at Complidata understand that data privacy is as precious to a financial institution as it is for an individual. We believe General Data Protection Regulation (GDPR) was promulgated in Europe because privacy is a precious value to us Europeans. It is hence of paramount importance for Complidata to avoid asking for the personal data of customers from FIs unless the case for a legitimate purpose is duly verified and processed by FI.
For some use cases where Complidata uses AI, it is inevitable to ask for customer information for example to optimize and reduce risk in Batch Screening of Customers or Sanctions Screening. While in other cases like Transaction Monitoring, the nominal details of customers are not requested.
Proportionate Use:
It is important to be able to monitor a risk governance solution built using AI. Complidata has built a model monitoring framework to allow FIs to monitor Machine Learning (ML) models both in pre-production and production phases. This monitoring allows the FIs to monitor bias and drift in the model and allow FIs to take necessary remediations to tackle them.
Design and Technical Expertise:
The challenge we faced as Complidata when working with FIs of various tiers is the limitations in skills of the non-data science staff in understanding the complexity of AI models. This involved them discarding the capacity of the AI models in tackling some issues normally dealt with by manual intervention. It also involved underestimating the risks involved in monitoring an AI model in production. While top-tier banks do have the capacity and mean to upskill their staff, it is not easy for most other FIs to set the standards for technical expertise. Learning from these experiences, we at Complidata strived hard to break the barriers of the complexity of AI models using tools like explainability, model monitoring, and automated retraining. We designed our solution to mitigate the risk of using AI and optimize the efficiency of the AI solutions irrespective of the technical expertise of the staff handling the AI solution in production.
Accountability and Oversight:
Despite the challenge of upskilling the entire AML organisation, it is still possible for FIs to involve their staff and make them responsible and accountable for managing AI models placed in production. This is usually done by deep knowledge transfer from the external model implementors like Complidata to the internal staff with well-documented training program and interactive sessions before the AI model is placed in production. It is also necessary to provide guidelines for model oversight and suggest good practices to monitor AI. Some examples include suggesting a quarterly retraining program for AI models to account for data drift if the capacity of FI in terms of workload to set up retraining is a maximum of 4 times per year. External assistance and oversight can also be suggested after the model is placed in production to limit the risk of drift and bias in AI.
Openness and Transparency:
Financial Crime AI models must be as open and explainable as they can, to be able to comply with regulatory requirements. This principle is achieved through detailed documentation of the various steps deployed in the Machine learning pipeline, writing clean, readable production-ready code for AI models in production, using version control systems to track and monitor changes, and using Machine Learning Operations (MlOps) tools like Kubeflow or MLFlow to manage and control ML workflows while working collaboratively. Complidata’s AI team for FCRR specializes in developing high-performance explainable AI solutions which not only cater to technical experts but also to domain experts using two levels of explanations. This level of transparency is essential to bridge the gap between domain experts, who face real-time challenges working with data at a micro level hands-on, and AI experts, who understand these challenges. Such tools improve the work efficiency of domain experts by providing them with explainable AI solutions. However, it is important to make sure this transparency does not breach the confidentiality requirements of ML reporting or limit the capabilities of AI. Although the transparency requirement limits the use of FCRR deep learning models for now, with the explainability aspect of deep learning models currently in active research, this may open the door to using explainable AI solutions using deep learning in the future for financial crime.
We at Complidata have a high-performing, well-tested, highly explainable, and highly experienced AI solution for Financial Crime Risk Reduction (FCRR) which is developed with the above principles in mind. We would stand by our principles to reduce risk in Financial Crime in an ethical, legally compliant, and very efficient way.